A Checklist for Native Advertising: How to Comply with the FTC’s New Rules
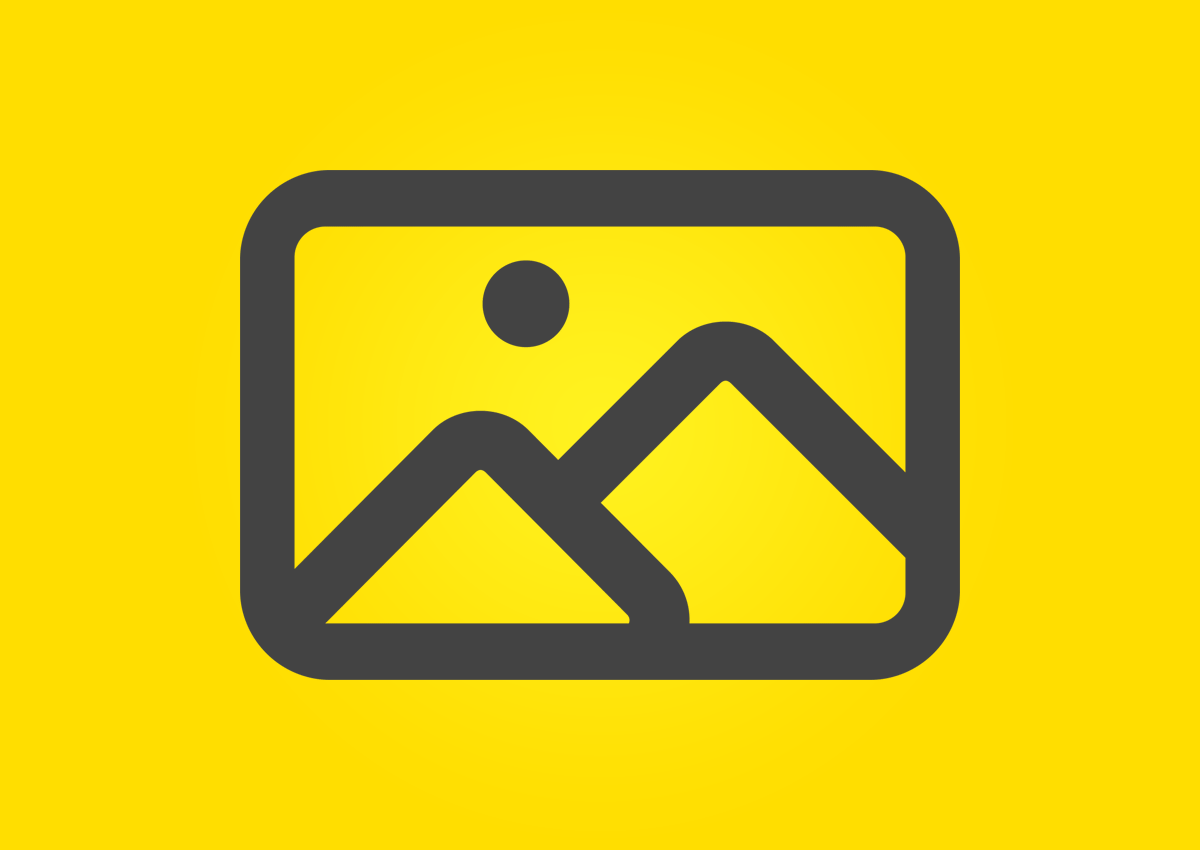
Posted by willcritchlow
The FTC recently published their updated rules (and more accessible “guidance”) on what constitutes a “misleading” native advert [PDF]. I’ve read them. I only fell asleep twice. Then, near the end, a couple of bombshells.
But first, the background.
Native ads and the FTC
For those who haven’t been following the trends closely, native advertising is a form of digital advertising whereby adverts are included “in-stream,” interspersed with regular editorial content.
On social platforms, this takes the form of “promoted” posts — including stories or videos in your Facebook stream or tweets in your Twitter stream from brands you didn’t explicitly follow or “Like.” See, for example, this Coursera ad in my Facebook stream:
Native ads are particularly interesting on mobile, where the smaller screens and personal nature tend to make anything that isn’t in-stream intrusive or irrelevant.
For publishers, native advertising looks more like brand content presented as whole pages rather than as banners or advertising around the edges of the regular content. It can take the form of creative content promoting the brand, brand-funded “advertorial,” or anything in between. See, for example, this well-labelled advertorial on Autocar:
You might notice that this is actually a lot like offline magazine advertising — where “whole page takeovers” are common, and presented in the “stream” of pages that you turn as you browse. And in a similar way to the digital version, they can be glossy creative or advertorial that looks more like editorial content.
The big way that the digital world differs, however, is in the way that you find content. Most people turn pages of a magazine sequentially, whereas a lot of visitors to many web pages come from search engines, social media, email, etc. — essentially, anywhere but the “previous page” on the website (whatever that would even mean).
It’s this difference that has led the FTC to add additional regulations on top of the usual ones banning misleading advertising — the new rules are designed to prevent consumers from being misled if they fail to realize that a particular piece is sponsored.
For the most part, if you understood the spirit of the previous rules, the new rules will come as no surprise — and the newest parts mainly relate to ensuring that consumers are fully aware why they are seeing a particular advert (i.e. because the advertiser paid for its inclusion) and they are clear on the difference between the advert and the editorial / unpaid content on the publisher’s site.
At a high level, it seems very reasonable to me — the FTC wants to see clear disclosures, and will assess confusion and harm in the context of consumers’ expectations, the ways in which confusion would cause them to behave differently, and will take into account the rest of the publisher’s site:
The Commission will find an advertisement deceptive if the ad misleads reasonable consumers as to its nature or source, including that a party other than the sponsoring advertiser is its source. Misleading representations of this kind are likely to affect consumers’ decisions or conduct regarding the advertised product or the advertisement, including by causing consumers to give greater credence to advertising claims or to interact with advertising content with which they otherwise would not have interacted.
And, crucially:
The FTC considers misleadingly formatted ads to be deceptive regardless of whether the underlying product claims that are conveyed to consumers are truthful.
They summarize the position as:
From the FTC’s perspective, the watchword is transparency. An advertisement or promotional message shouldn’t suggest or imply to consumers that it’s anything other than an ad.
Subjectivity
I was interested to see the FTC say that:
Some native ads may be so clearly commercial in nature that they are unlikely to mislead consumers even without a specific disclosure.
While I think this would be risky to rely upon without specific precedents, it nicely shows more of the FTC’s intent, which seems very reasonable throughout this briefing.
Unfortunately, the subjectiveness cuts both ways, as another section says:
“…the format of [an] advertisement may so exactly duplicate a news or feature article as to render the caption ‘ADVERTISEMENT’ meaningless and incapable of curing the deception.”
It’s not easy to turn this into actionable advice, and I think it’s most useful as a warning that the whole thing is very subjective, and there is a lot of leeway to take action if the spirit of the regulations is breached.
The controversial and unexpected parts
It wasn’t until quite far through the document that I came to pieces that I found surprising. The warning bells started sounding for me when I saw them start drawing on the (very sensible) general principle that brands shouldn’t be able to open the door using misleading or deceptive practices (even if they subsequently come clean). Last year, the FTC took action against this offline advert under these rules:
Ruling that the price advertised in big red font was a “deceptive door opener” because:
To get the advertised deal, buyers needed to qualify for a full house of separate rebate offers. In other words, they had to be active duty members of the military and had to be recent college grads and had to trade in a car.
Bringing it back to web advertising, the Commission says:
Under FTC law, advertisers cannot use “deceptive door openers” to induce consumers to view advertising content. Thus, advertisers are responsible for ensuring that native ads are identifiable as advertising before consumers arrive at the main advertising page. [Emphasis mine]
If you understand how the web works, and how people find content on publishers’ sites these days, this will probably be starting to seem at odds with the way a lot of native advertising works right now. And your instincts are absolutely right. The Commission is going exactly where you think they might be. They title this set of new rules “Disclosures should remain when native ads are republished by others.”
Social media
In the guidelines document, the Commission includes a whole bunch of examples of infringing and non-infringing behavior. Example 15 in their list is:
The … article published in Fitness Life, “Running Gear Up: Mistakes to Avoid,” … includes buttons so that readers can post a link to the article from their personal social media streams. When posted, the link appears in a format that closely resembles the format of links for regular Fitness Life articles posted to social media. In this situation, the ad’s format would likely mislead consumers to believe the ad is a regular article published in Fitness Life. Advertisers should ensure that the format of any link for posting in social media does not mislead consumers about its commercial nature. [Emphasis mine]
Now, it’s obviously really hard to ensure anything about how people post your content to social media. In the extreme case, where a user uses a URL shortener, doesn’t use your title or your Open Graph information, and writes their own caption, there could be literally nothing in the social media post that is in the control of the advertiser or the publisher. Reading this within the context of the reasonableness of the rest of the FTC advice, however, I believe that this will boil down to flagging commercial content in the main places that show up in social posts.
Organic search
Controlling the people who share your content on social media is one challenge, but the FTC also comments on the need to control the robots that display your content in organic search results, saying:
The advertiser should ensure that any link or other visual elements, for example, webpage snippets, images, or graphics, intended to appear in non-paid search results effectively disclose its commercial nature.
…and they also clarify that this includes in the URL:
URL links … should include a disclosure at the beginning of the native ad’s URL.
Very sensibly, it’s not just advertisers who need to ensure that they abide by the rules, but I find it very interesting that the one party noticeably absent from the FTC’s list is the publishers:
In appropriate circumstances, the FTC has taken action against other parties who helped create deceptive advertising content — for example, ad agencies and operators of affiliate advertising networks.
Historically, the FTC has maintained that it has the authority to regulate media companies over issues relating to misleading advertising, but has generally focused on the advertisers; for example, when talking about taking special measures to target a glut of misleading weight loss adverts:
“…the FTC said it does not plan to pursue media outlets directly, ‘but instead wants to continue to work with them to identify and reject ads with obviously bogus claims’ using voluntary guidelines.”
In the case of native advertising, I am very surprised not to see more of the rules and guidelines targeted at publishers. Many of the new rules refer to platform and technical considerations, and elements of the publishers’ CMS systems which are likely to be system-wide and largely outside the control of the individual advertisers. Looking at well-implemented native ads released prior to these new guidelines (like this one from the Telegraph which is clearly and prominently disclosed), we see that major publishers have not been routinely including disclosures in the URL up to now.
In addition, individual native ads could remain live and ranking in organic search for a long time, yet the publisher could undergo redesigns / platform changes that change things like URL structures. I doubt we’d see this pinned on individual advertisers, but it is an interesting wrinkle.
Checklist for compliant native advertising
Clearly I’m not a lawyer, and this isn’t legal advice, but from my reading of the new rules, advertisers should already have been expecting to:
- Ensure you comply with all the normal rules to ensure that your advert is not misleading in content including being sure to avoid “deceptive door openers”
- “Clearly and prominently disclose the paid nature” of native adverts — it is safest to use language like “Advertisement” or “Paid Advertisement” — and this guide has detailed guidance.
In addition, following the release of these new rules, advertisers should also work through this checklist:
- The URL includes a disclosure near the beginning (e.g. example.com/advertisement/<slug>)
- The title includes a disclosure near the beginning
- The meta description includes a disclosure
- All structured data on the page intended to appear in social sharing contains disclosures:
- Open Graph data
- Twitter cards
- Social sharing buttons’ pre-filled sharing messages
- Given the constraints on space in tweets especially, I would suggest this could be shorter than in other places — a simple [ad] probably suffices
- Links to the native advert from elsewhere on the publisher’s site includes disclosures in both links and images
- Embedded media have disclosures included within them — for example, via video and image overlays
- Search engines can crawl the native advert (if it’s blocked in robots.txt, the title tag and meta description disclosures wouldn’t show in organic search)
- Outbound links are nofollow (this is a Google requirement rather than an FTC one, but it seemed sensible to include it here)
And it seems sensible to me that advertisers would require publishers to commit to an ongoing obligation to ensure that their CMS / sharing buttons / site structure maintains compliance with the FTC regulations for as long as the native advertising remains live.
Conclusion
There are elements of the new requirements that seem onerous, particularly the disclosure early in the URL which could be a technically complicated change depending on the publisher’s platform. I have looked around at a bunch of major publisher platforms, and I haven’t found a high-profile example that does disclose all advertorials in their URLs.
It also seems likely that full compliance with all these requirements will reduce the effectiveness of native advertising by limiting its distribution in search and social.
On balance, however, the FTC’s approach is based in sound principles of avoiding misleading situations. Knowing how little people actually read, and how blind we all are to banners, I’m inclined to agree that this kind of approach where the commercial relationship is disclosed at every turn probably is the only way to avoid wide-scale misunderstandings by users.
I’d be very interested to hear others’ thoughts. In particular, I’d love to hear from:
- Brands: Whether this makes you less likely to invest in native advertising
- Publishers: Whether these technical changes are likely to be onerous and if it feels like a threat to selling native ads
I look forward to hearing your thoughts in the comments.
Sign up for The Moz Top 10, a semimonthly mailer updating you on the top ten hottest pieces of SEO news, tips, and rad links uncovered by the Moz team. Think of it as your exclusive digest of stuff you don’t have time to hunt down but want to read!
Continue reading →