IPv6, C-Blocks, and How They Affect SEO
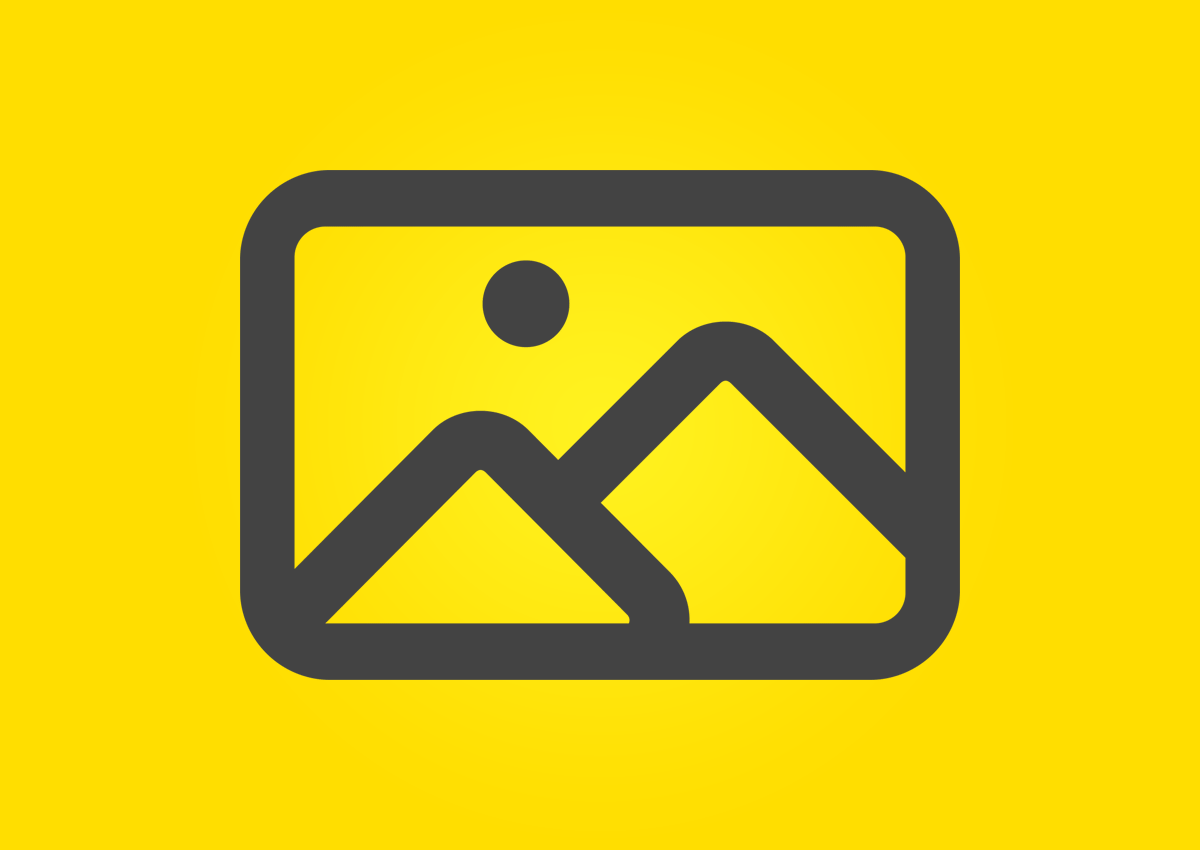
Posted by Tom-Anthony
You have probably heard about IPv6, but you might remain a bit confused about the details of what it is, how it works, and what it means for the future of the Internet. This post gives a quick introduction to IPv6, and discusses the SEO implications that could follow from the IPv6 roll-out (touching specifically on the concept of C-Blocks). A quick caveat: This stuff is hard, so let me know if you spot any missteps!
A very brief intro to IP addresses (v4) & c-blocks
Example IP address (IPv4).
This format of an IP address is the common format in use everywhere, and is called IPv4. There are four bytes in an IP address like this, with each byte separated by a period (meaning 32 bits in total, for the geeks). Every (sub)-domain resolves to at least one such IP address (it might be several, but lets ignore that for now). Nice and simple.
So why is this interesting to us? Why is this important to SEO? The old-school logic is that if you have two IPs that are in the same C-Block, then the sites are quite likely related and thus the links between these sites (on average) should not count as strongly in terms of PageRank. My personal opinion is that nowadays there are many many other signals available to Google to make these same sorts of connections and so the C-Block issue is far less important than it once was.
Disney and ABC have a near identical IP address, both in the same C-Block.
Introducing IPv6
So, there is a problem with IP addresses in the format above (IPv4); there are “only” 4 billion of them, and we have essentially exhausted the supply. We have so many connected devices nowadays, and the creators for IPv4 never envisioned the vastness of the Internet 30 years from when it was released. Luckily enough, they saw the problem early on andstarted working on a successor, IPv6 (IPv5 was used for another unreleased protocol).
IPv6 address format:
An example IPv6 address.
Things just got serious! There are now 8 blocks rather than 4, and rather than each block being 1 byte (which were represented as a number from 0-255), each block is instead 2 bytes represented by 4 hexadecimal characters. There are 128 bits in an IPv6 address, meaning instead of a measly 4,000,000,000 like IPv4, IPv6 has around 340,000,000,000,000,000,000,000,000,000,000,000,000 addresses.
CIDR Notation
Before we go any further, it is important to introduce an important concept for understanding IP addresses, which is called CIDR notation.
IPv6 exclusively uses CIDR notation (e.g. /24), so the SEO community will need to understand this concept. It is really simple, but normally really badly explained.
Example IPv4 IP address shown in dot decimal format and as binary.
Two IP addresses in the same C-Block. The first 24 bits (3 blocks of 8 bits) are identical.
This can be represented in this case as 199.181.132/24.
Now CIDR notation is more refined and more accurate than the concept of C-Block; in the example above the two IP addresses are not just in the same C-Block they are even more closely related as 6 bits in the last block are also identical. In CIDR notation we could say both these IP addresses are in the 199.181.132/30 block to indicate that the 30 leading bits are identical.
IPv6 & C-Blocks?
Now CIDR /24 is not exactly catchy and so someone made up the name “C-Block” to make this easier to talk about, but it doesn’t extend so easily to IPv6. So, the question is, can we generalise something similar?
The point of a C-Block from Google’s perspective and the perspective of our SEO is solely to identify whether links are originating on the same ISP network. So that should obviously remain the focus. So my best guess would be to focus on how these IPs are allocated to ISPs (ISPs normally get large continuous blocks of IP addresses they can then use for their customers’ websites).
Illustration of an “ISP Block” (/32); the blue part of the address is stable and
indicates the ISP. The red part can change and represents addresses at that ISP.
With IPv6, I believe that ISPs will be given /32 blocks (the leading 32 bits will be the same, leaving 96 bits to create addresses for their customers), which they will then assign to their users in /64 blocks (I asked a few people, this tends to be what is happening, but I have read that this might sometimes be /48 blocks instead). Notice that ISPs now have an order of magnitude more IP addresses (each) than the whole internet had before!
This also means each end user will get more IP addresses for their own network than there are in total IPv4 IP addresses. Welcome to the Internet of things!
Illustration of a “Customer Block” (/64); the blue part indicates a particular customer.
The red part can change and represents addresses belonging to that customer.
So, I think the equivalent of a C-Block in IPv6 land would be a /32 block because that is what an ISP will usually be assigned (and allows them to then carve that up into 4 billion /64 blocks for their users!).
Furthermore, in IPv6 the minimum allocation is /32 so a single /32 block cannot run across multiple ISPs as I understand it, so there is no way two IPs in the same /32 could belong to two different ISPs. If our goal is to continue to examine whether sites are more likely related than two random sites, then knowing they are on the same ISP (which is what C-Blocks do) is our goal.
Also, if you chose /64 then each ISP has 4 billion of these blocks to give away, and that is way too sparse to identify associations between sites in different blocks.
Geek side note: indeed, the “host” http header accepts an IPv6 address to distinguish which site on the server you want.
So now a single server with multiple sites will have a separate IP for each of those sites (it is also possible that the server has multiple IPv6 blocks assigned, one for each different customer – I think this is actually the intention and hopefully becomes the reality).
My recommendation on an IPv6 C-Block
- Sites in the same /32 block as before would be equivalent to the same C-Block as previously.
- Sites in the same /64 block either are on the exact same server, or belong to the same customer, so are even closer related than C-Block level.
- “ISP Block” for /32 blocks.
- “Customer Block” for /64 blocks.
- In IPv6 IP addresses in the same ISP Blocks most closely resemble the relationship of IPs in the same C-Block in IPv4.
- In IPv6 IP addresses in the same User Block are likely very closely related, and probably belong to the same person/organisation.
What should I take away from all this?
With IPv6, I think the “Customer Blocks” could be a very important SEO feature, as it is an even closer relationship than C-Blocks were, and this is something that Google will likely make use of. It is still going to take a while until IPv6 becomes prevalent enough that all of this is important, so for the moment this is just something to have on your radar as it will begin to increase in importance over the next couple of years.
Sign up for The Moz Top 10, a semimonthly mailer updating you on the top ten hottest pieces of SEO news, tips, and rad links uncovered by the Moz team. Think of it as your exclusive digest of stuff you don’t have time to hunt down but want to read!
Continue reading →