Behind the Scenes of Fresh Web Explorer
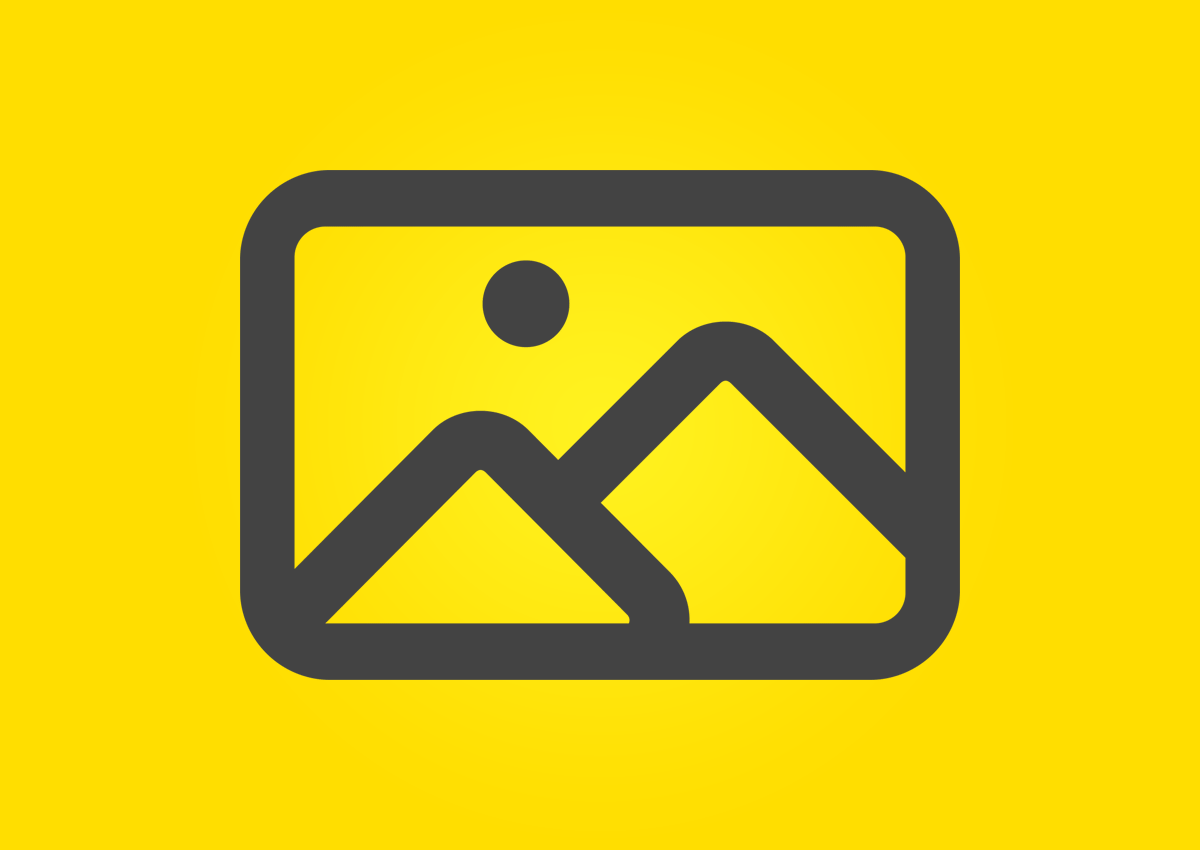
Posted by dan.lecocq
Fresh Web Explorer is conceptually simple — it’s really just a giant feed reader. Well, just a few million of what we think are the most important feeds on the web.
At a high level, it’s arranged as a pipeline, beginning with crawling the feeds themselves and ending with inserting the crawled data into our index. In between, we filter out URLs that we’ve already seen in the last few months, and then crawl and do a certain amount of processing. Of course, this wouldn’t be much of an article if it ended here, with the simplicity. So, onwards!
The smallest atoms of work that the pipeline deals with is a job. These are pulled off of various queues by a fleet of workers, processed, and then handed off to other workers. Different stages take different amounts of times, are best suited to certain types of machines, and thus it makes sense to use queues in this way. Because of the volume of data that must move through the system, it’s impractical to pass the data along with each job. In fact, workers are frequently uploading to and downloading from S3 (Amazon’s Simple Storage Service) and just passing around references to the data stored there.
The queueing system itself is one we talked about several months ago called “qless.” Fresh Web Explorer is actually one of the two projects for which qless was written (campaign crawl’s the other), though it has found adoption for other projects from our data science team to other as-of-yet announced projects. Here’s an example of what part of our crawl queue looks like, for example:

In each of the following sections, I’ll be talk about some of the hidden challenges to many of these seemingly-innocuous stages of the pipeline, as well as the particular ways in which we’ve tackled them. To kick this process off, we begin with the primordial soup out of which this crawl emerges: the schedule of our feeds to crawl.
Scheduling
Like you might expect on the web, a few domains are responsible for most of the feeds that we crawl. Domains like Feedburner and Blogspot come to mind, in particular. This becomes problematic in terms of balancing politeness with crawling in a reasonable timeframe. For some context, our goal is to crawl every feed in our index roughly every four hours, and yet some of these domains have hundreds of thousands of feeds. To make matters worse, this is a distributed crawl on several workers, and coordination between workers is severely detrimental to performance.
With job queues in general, it’s important to strike a balance between too many jobs and jobs that take too long. Jobs sometimes fail and must be retried, but if the job represents too much work, a retry represents a lot of wasted work. Yet, if there are too many jobs, the queueing system becomes inundated with operations about maintaining the state of the queues.
To allow crawlers to crawl independently and not have to coordinate page fetches with one another, we pack as many URLs from one domain as we can into a single job subject to the constraint that it could be crawled in a reasonable amount of time (on the order of minutes, not hours). In the case of large domains, fortunately, the intuition is that if they’re sufficiently popular on the web, then they can handle larger amounts of traffic. So we pack all these URLs into a handful of slightly larger-than-normal jobs in order to limit the parallelism, and so long as each worker obeys politeness rules, we’re guaranteed a global close approximation to politeness.
Deduping URLs
Suffice it to say, we’re reluctant to recrawl URLs repeatedly. To that end, one of the stages of this pipeline is to keep track of and remove all the URLs that we’ve seen in the last few months. We intentionally kept the feed crawling stage simple and filter-free, and it just passes _every_ url it sees to the deduplication stage. As a result, we need to process hundreds of millions of URLs in a streaming fashion and filter as needed.
As you can imagine, simply storing a list of all the URLs we’ve seen (even normalized) would consume a lot of storage, and checking would be relatively slow. Even using an index would likely not be fast enough, or small enough, to fit on a few machines. Enter the bloom filter. Bloom filters are probabilistic data structures that allow you to relatively compactly store information about objects in a set (say, the set of URLs we’ve seen in the last week or month). You can’t ask a bloom filter to list out all the members of the set, but it does allow you to add and query specific members.
Fortunately, we don’t need to know all the URLs we’ve seen, but just answer the question: have we seen _this_ url or _that_ url. A couple of downsides to bloom filters: 1) they don’t support deletions, and 2) they do have a small false positive rate. The false positive rate can be controlled by allocating more space in memory, and we’ve limited ours to 1 in 100,000. In practice, it turns out to often be less than that limit, but it’s the highest rate we’re comfortable with. To get around the lack of being able to remove items from the set, we must resort to other tricks.
We actually maintain several bloom filters; one for the current month, another for the previous month, and so on and so forth. We only add URLs that we’ve seen to the current month, but when filtering URLs out, we check each of the filters for the last _k_ months. In order to allow these operations to be distributed across a number of workers, we use an in-memory (but disk-backed) database called Redis and our own Python bindings for an in-Redis bloom filter, pyreBloom. This enables us to filter tens of thousands of URLs per second and thus, keep pace.
Crawling
We’ve gone through several iterations of a Python-based crawler, and we’ve learned a number of lessons in the process. This subject is enough to merit its own article, so if you’re interested, keep an eye on the dev blog for an article on the subject.
The gist of it is that we need a way to efficiently fetch URLs from many sources in parallel. In practice for Fresh Web Explorer, this is hundreds or thousands of hosts at any one time, but at peak it’s been on the order of tens of thousands. Your first instinct might be to reach for threads (and it’s not a bad instinct), but it comes with a lot of inefficiencies at the expense of conceptual simplicity.
There are mechanisms for the ever-popular asynchronous I/O that are relatively well-known. Depending on what circles in which you travel, you may have encountered some of them. Node.js, Twisted, Tornado, libev, libevent, etc. At their root, these all use two main libraries: kqueue and epoll (depending on your system). The trouble is that these libraries expose a callback interface that can make it quite difficult to keep code concise and straightforward. A callback is a function you’ve written that you give to a library to run when it’s done doing it’s processing. It’s something along the lines of saying, ‘fetch this page, and when you’re done, run this function with the result.’ While this doesn’t always lead to convoluted code, it can all too easily lead to so-called ‘callback hell.’
To our rescue comes threading’s lesser-known cousin, coroutines and incarnated in gevent. We’ve tried a number of approaches, and in particular we’ve been burned by the aptly-named “twisted.” Gevent has been the sword that has cut the gordian knot of crawling. Of course, it’s not a panacea, and we’ve written a lot of code to help make common crawling tasks easy. Tasks like URL parsing and normalization, and robots.txt parsing. In fact, the Python bindings for qless even have a mode that is gevent-compatible, so we can still keep our job code simple and still make full use of gevent’s power.
A few crawlers is actually all it takes to maintain steady state for us, but we’ve had periods where we wanted to accelerate crawling (for backlogs, or to recrawl when experimenting). By way of an example of the kind of power the coroutines offer, here are some of our crawl rates for various status codes scaled down to 10%. This graph is from a time when we were using 10 modestly-sized machines, and while maintaining politeness they sustain about 1250 URLs/second including parsing, which amounts to about 108 million URLs a day at a cost of about $1 per million. Of course, this step alone is just a portion of the work that goes into making Fresh Web Explorer.
Dechroming
There’s a small amount of processing associated with our crawling. Parse the page, look at some headers, et. all, but the most interesting feature of this process is the dechroming: trying to remove all the non-content markup in a page, from sidebars to headers to ads. It’s a difficult task, and no solution will be perfect. Despite that, through numerous hours and great effort (the vast majority of which has been provided by our data scientist, Dr. Matt Peters) we have a reasonable approach.
Dechroming is an area of active research in certain fields, and there are certainly some promising approaches. Many of the earlier approaches (including that of blogscape from our tools section, Fresh Web Explorer’s predecessor) relied on finding many examples from a given site, and then using that information to try to find the common groups of elements. This has the obvious downside of needing to be able to quickly and easily access other examples from any given site at any given time. Not only this, but it’s quite sensitive to changes to website markup and changes in chrome.
Most current research focuses instead on finding a way to differentiate chrome from content with a single page example. We actually began our work by implementing a couple of algorithms described in papers. Perhaps the easiest to conceptually understand is one in which a distribution of the amount of text per block (this doesn’t have a 1:1 correspondence with HTML tags, necessarily) and then finding the clumps within that. The intuition is that the main content is likely to be larger sequential blocks of text than, say, comments or sidebars. In the end, our approach ended up being a combination of several techniques and you can find out more about it in our “dragnet” repo.
All told
Fresh Web Explorer has been in the works for a long while — perhaps longer than I’d care to admit. It has been rife with obstacles overcome (both operational and algorithmic) and lessons learned. These lessons will be carried forward in subsequent iterations and future projects. There are many changes we’d like to make given this hindsight and of course we will. Refactoring and maintaining code is often more time-consuming than writing the original!
The feedback from our community has generally been positive so far, which is encouraging. Obviously we hope this is something that will not only be useful, but also enjoyable for our customers. The less-than-positive feedback has highlighted some issues of which we are aware, most of which are high on our priorities, and leaves us raring to go to make it better.
On many points here there are many equally valid approaches. While time and space don’t permit us to present a complete picture, we’ve tried to pull out the most important parts. If there are particular questions you have about other aspects of this project or why we chose to tackle an issue one way or another, please comment! We’re happy to field any thoughts you might have on the subject 🙂
Sign up for The Moz Top 10, a semimonthly mailer updating you on the top ten hottest pieces of SEO news, tips, and rad links uncovered by the Moz team. Think of it as your exclusive digest of stuff you don’t have time to hunt down but want to read!